Within TKI Water Technology’s Smart Water Systems theme, the drinking water company Brabant Water, working with three knowledge partners, has smartly combined data from various sources and parameters with big data techniques. The DiAMANT-Water project demonstrates that data-driven analysis is a useful complement to the investigation of physical processes: two detailed cases show, among other things, that brown water correlates with temperature, that noise complaints about water meters correlate with the house’s construction date, and failures of pipes containing cement correlate with large pressure variations over the course of the day. There is however no ready-to-use recipe: Knowledge Discovery in Databases (KDD) needs to be tailored to each case.
Drinking water companies are making increasing use of online sensors, automation and computerisation in their production and distribution activities. They are therefore generating ever-larger volumes of ‘water’ data. At the same time there is a growing supply of open data: publicly accessible datasets produced by a variety of organisations – for instance, data on the demographic properties of public space, or datasets that include ambient temperature and groundwater levels. With the Knowledge Discovery in Databases (KDD) analysis method it is often possible to distil valuable knowledge from the combinations of such data sources. This knowledge can then be applied to improve information collection and decision-making at the operational level. Drinking water company Brabant Water wanted to know whether it could improve its drinking water supply operations through data mining and KDD. A consortium comprising KWR, Nelen and Schuurmans, and Witteveen+Bos elaborated two cases for this purpose. KWR carried out a proof-of-principle on the introduction and utility of the techniques for the two cases; Nelen and Schuurmans visualized the data and model results using the Lizard™ platform; and Witteveen+Bos played an advisory role.
Customer report correlations
The first case studied how KDD can be applied to customer reports, distribution net data, buildings, ambient temperature and the demographics of neighbourhoods and districts. This revealed a correlation between temperature and the number of reports of brown water, and a correlation between noise complaints about water meters in homes and the building’s year of construction: an indication that the location of a water meter in a home can be of key importance.
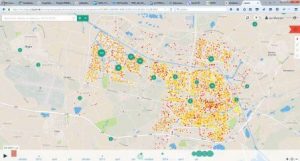
Figure: Combined visualization of different data sources in LizardTM : water consumption in yellow-orange-red (from low to high) and clustered client reports of noise nuisance (green circles with number of reports in the middle).
Failures correlated to pressure differences
The second case studied whether KDD can be used to draw on failure registration information, real-time data from the process information system (flow-pressure and -volume data), and distribution network information to gain insight into and test hypotheses about failure frequencies. Among other things this revealed that pipes containing cement fail more frequently when pressure levels vary greatly, while other pipes fail mostly under normal pressure variations.
Useful complement
Data-driven analysis is a useful complement to the investigation of physical processes, for instance as an initial screening of multiple possible explanations which require closer (physical) investigation. KDD can also help extract usable parameters from high-frequency measurements and from customer reports, in which visualization and support with statistical methods make an important contribution. Such data management, sector-wide data sharing (about failures and reports, as well as operational data), and data visualization can support the use of KDD and statistics and accelerate the analysis. Knowledge about the distribution network and about how the information is collected, aggregated and combined is essential for the effective implementation of KDD and data mining: tailored work is required, there is no ready-to-use recipe.